Land Price Prediction with Machine Learning in Mueang Khon Kaen District
DOI:
https://doi.org/10.14456/jcct.2024.8Keywords:
Land Price Assessment, Machine Learning, Important FactorsAbstract
This research aims to develop a model of land price assessment and study of factors influencing land prices with machine learning used as a guideline for determining the estimated price to be close to the actual purchase price in Mueang Khon Kaen District from land price information traded on websites in enforcement department of 193 locations, and land price assessment information in land department of 1,500 locations in this study the factors involved include appraisal value, property type, land size, distance, and the average appraisal value of five nearby plots of land. The models used for analysis are Regression Tree, Random Forest, Gradient Boosted Trees, and Linear Regression. Which, the land price assessment from the case study by model measurement in MAE, RMSE, R-squared, Grid Search, and Cross-validation to selected model parameters and evaluate their performance. The results found that the model with the best predictive performance is Gradient Boosted Trees in R-squared at the highest of 0.80, MAE, and RMSE at the lowest of 7929.40, and 15281.33, respectively. Feature Importance in the locations with the most influence on prediction, followed by area size, average appraised value from five nearby locations, and property type.
Downloads
References
Bergstra, J., & Bengio, Y. (2012). Random Search for Hyper-Parameter Optimization. Journal of Machine Learning Research, 13(10), 281–305.
Burnham, K. P., & Anderson, D. R. (2002). Model Selection and Multimodel Inference: a Practical Information-Theoretic Approach. Springer Science & Business Media.
Chandrashekar, G., & Sahin, F. (2014). A Survey on Feature Selection Methods. Computers & Electrical Engineering, 40(1), 16–28. https://doi.org/10.1016/j.compeleceng.2013.11.024.
Choompol, A. (2019). Feature Selection and Redundant Feature Elimination for Opinion Classification on Social Network. [Doctoral dissertation, Mahasarakham University]. Mahasarakham University Intellectual Repository. http://202.28.34.124/dspace/handle/123456789/537. (In Thai).
Guyon, I., & Elisseeff, A. (2003). An Introduction to Variable and Feature Selection. Journal of Machine Learning Research, 3(7–8), 1157–1182.
Kohavi, R. (1995). A Study of Cross-Validation and Bootstrap for Accuracy Estimation and Model Selection. Proceedings of the 14th International Joint Conference on Artificial Intelligence - Volume 2, 1137–1143.
Kumpu, P., & Piyathamronchai, K. (2024). Application of the Geographic Information System to Devolop Land Valuation Models, Case Study: Muang Chiangmai District, Chiangmai Province. The Journal of Spatial Innovation Development, 5(2), 42–64. (In Thai)
Lundberg, S., & Lee, S.-I. (2017). A Unified Approach to Interpreting Model Predictions. 31st Conference on Neural Information Processing Systems. 4765-4774. https://doi.org/10.48550/ARXIV.1705.07874.
Na Bangchang, K. (2011). A Variable Selection in Multiple Linear Regression Models Based on Tabu Search [National Institute of Development Administration]. https://doi.org/10.14457/NIDA.the.2011.17. (In Thai).
Pedregosa, F., Varoquaux, G., Gramfort, A., Michel, V., Thirion, B., Grisel, O., Blondel, M., Müller, A., Nothman, J., Louppe, G., Prettenhofer, P., Weiss, R., Dubourg, V., Vanderplas, J., Passos, A., Cournapeau, D., Brucher, M., Perrot, M., & Duchesnay, É. (2012). Scikit-learn: Machine Learning in Python. Journal of Machine Learning Research, 12, 2825–2830. https://doi.org/10.48550/ARXIV.1201.0490
Soltani, A., Heydari, M., Aghaei, F., & Pettit, C. J. (2022). Housing Price Prediction Incorporating Spatio-Temporal Dependency into Machine Learning Algorithms. Cities, 131, 103941. https://doi.org/10.1016/j.cities.2022.103941.
Vanderplas, J. (2017). Python Data Science Handbook: Essential Tools for Working with Data. O’Reilly.
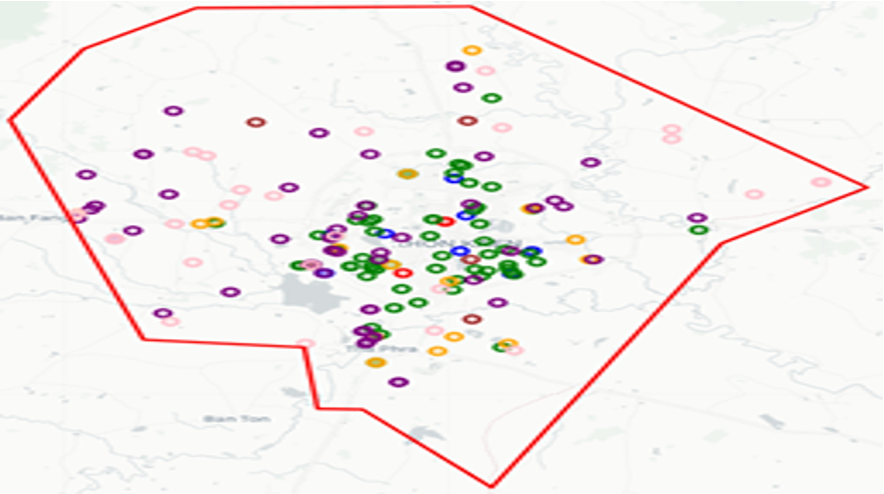
Downloads
Published
How to Cite
Issue
Section
Categories
License
Copyright (c) 2024 Journal of Computer and Creative Technology

This work is licensed under a Creative Commons Attribution-NonCommercial-NoDerivatives 4.0 International License.