Analyze the Chonburi Provincial Tourism Authority using Data Mining Methods
DOI:
https://doi.org/10.14456/jcct.2024.13Keywords:
Tourist Behavior, Tourist Attractions, Chonburi Province, Data Mining TechniquesAbstract
Chonburi province has a variety of tourist attractions that make some tourists unable to decide which tourist attractions to choose that meet their needs. This research aims to analyze the behavior and factors affecting the decision-making of tourism in Chonburi Province by data mining methods to create a model for recommending tourist attractions from data collection using questionnaires with 120 samples of local tourists. Data analysis using four data mining techniques including Naïve Bayes, Decision Tree, K-Nearest Neighbors, and Multi-Layer Perceptron. In this regard, the study findings on factors that have a high influence on the decision to choose a tourist destination include nature preference, relaxation, and convenience of travel on the framework for developing and creating applications or platforms to recommend tourist attractions that are consistent with and meet the needs of future tourists effectively.
Downloads
References
Bunrueang, S. (2019). Development of Tourism Potential in Chonburi Province for Sustainable Development. Review of Integrative Business and Economics Research, 8(3), 258-265.
Chancharat, S. (2011). Thai Tourism and Economic Development: The Current State of Research. Kasetsart Journal of Social Sciences, 32(2), 340–351.
Chaturongakul, D. (2022). An Analysis of the Factors Motivating Tourist Travel to Pattaya City, Chonburi Province, Under the Covid 2019 Pandemic (Covid-19). Humanities and Social Science Research Promotion Network Journal, 5(2), 1–16. (In Thai)
Chonburi Province. (n.d.). Get to know Chonburi. https://www.chonburi.go.th/content/profile. (In Thai)
Chutipascharoen, A., & Sanrach, D. C. (2018). A Comparison of the Efficiency of Algorithms and Feature Selection Methods for Predicting the Success of Personal Overseas Money Transfer. KKU Research Journal (Graduate Studies) Humanities and Social Sciences, 6(3), 105–113. (In Thai)
Edensor, T. (2000). Staging Tourism: Tourists as Performers. Annals of Tourism Research, 27(2), 322–344. https://doi.org/10.1016/S0160-7383(99)00082-1.
KongRuksiam Studio. (2020). K-Nearest Neighbors. https://kongruksiam.medium.com/สรุป-machine-learning-ep-4-เพื่อนบ้านใกล้ที่สุด-k-nearest-neighbors-787665f7c09d. (In Thai)
Mekparyup, J. & Saithanu, K. (2009). Application of Neural Networks to Multivariate Quality Control Charts. Burapha Science Journal, 14(2), 99-110.
Poolpol, P. (2021). Naïve Bayes Classification. https://peachapong-poolpol.medium.com/naïve-bayes-classification-cb6cf905505d. (In Thai)
Saxon, S., Sodprasert, J., & Sucharitakul, V. (2021). Reimagining Travel: Thailand Tourism after the COVID-19 Pandemic. https://www.mckinsey.com/industries/travel-logistics-and-infrastructure/our-insights/reimagining-travel-thailand-tourism-after-the-covid-19-pandemic.
Tourismatbuu. (n.d.). Meaning of Tourism. https://tourismatbuu.wordpress.com/ความรุ้เบื้องต้นการท่อ/ความหมายของการท่องเที่. (In Thai)
Utthayan, C. (2008). Meaning of Tourist Behavior. https://touristbehaviour.wordpress.com/1. (In Thai)
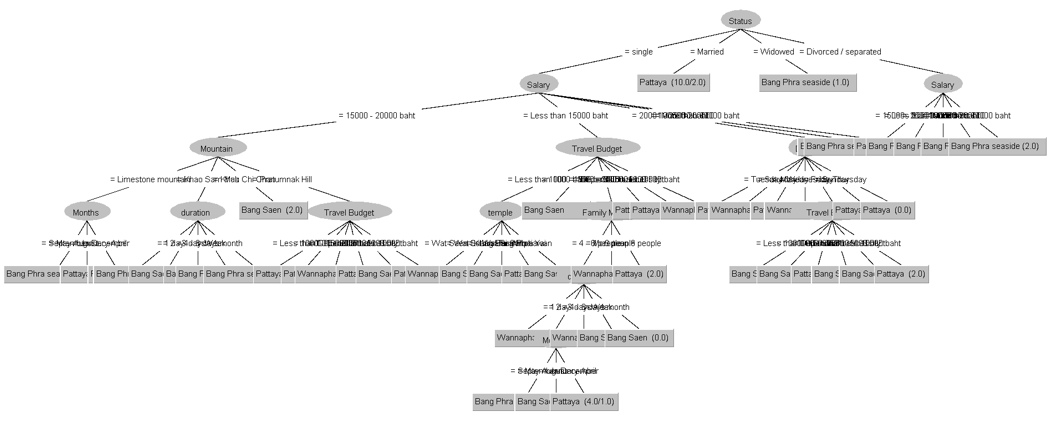
Downloads
Published
How to Cite
Issue
Section
Categories
License
Copyright (c) 2024 Journal of Computer and Creative Technology

This work is licensed under a Creative Commons Attribution-NonCommercial-NoDerivatives 4.0 International License.