Voice Vitalize: A Personalized Speech Rehabilitation Application for Stroke Patients
DOI:
https://doi.org/10.14456/jcct.2025.6Keywords:
Speech Rehabilitation, Stroke, Elderly, Speech-to-text Technology, Personalized Rehabilitation PlanAbstract
VoiceVitalize is a speech rehabilitation application designed for individuals with communication difficulties, particularly stroke patients and elderly people with unclear or impaired speech. This study aimed to develop and evaluate the effectiveness of the application, which utilizes speech-to-text technology and measures speech accuracy using Word Error Rate (WER). The research tools included a prototype application, user satisfaction questionnaires, and speech training logs. The tool was pre-tested by five experts and users before the main trial. Data were collected from 20 participants and analyzed using quantitative methods, comparing WER before and after application use, and qualitative analysis from open-ended questionnaire responses. The results showed significant improvement in participants’ speech. In Level 1 sentences, the WER decreased from 83.00% to 50.00%, and in Level 2 sentences, from 54.17% to 41.67% within 7 days. User feedback indicated that the application was user-friendly, helped reduce travel and healthcare costs, and was suitable for independent daily speech practice.
Downloads
References
Alharbi, S., Alrazgan, M., Alrashed, A., Alnomasi, T., Almojel, R., Alharbi, R., Alharbi, S., Alturki, S., Alshehri, F., & Almojil, M. (2021). Automatic Speech Recognition: Systematic Literature Review. IEEE Access, 9, 131858–131876. https://doi.org/10.1109/ACCESS.2021.3112535.
Barberis, M., Clercq, P. D., Tamm, B., hamme, H. V., & Vandermosten, M. (2024). Automatic Recognition and Detection of Aphasic Natural Speech. ArXiv. https://doi.org/10.48550/arXiv.2408.14082.
Errattahi, R., El Hannani, A., & Ouahmane, H. (2018). Automatic Speech Recognition Errors Detection and Correction: A Review. Procedia Computer Science, 128, 32–37. https://doi.org/10.1016/j.procs.2018.03.005.
Lee, S.-H., Kim, J., & Kim, H.-J. (2025). Smartphone Application–Based Voice and Speech Training Program for Parkinson Disease: Feasibility and Satisfaction Study with a Preliminary Rater-Blinded Single-Arm Pretest and Posttest Design. Journal of Medical Internet Research, 27, e63166. https://doi.org/10.2196/63166.
Massawe, R. (2024). Understanding and Calculating Word Error Rate (WER) in Automatic Speech Recognition using Python. Medium. https://medium.com/@ramadhanimassawe14/understanding-and-calculating-word-error-rate-wer-in-automatic-speech-recognition-using-python-661f18b518a5.
Office of Disease Prevention and Control Region 12 Songkhla. (2020). October 29: World Stroke Day - Collaborate to Prevent and Reduce the Risk of Paralysis. Department of Disease Control. https://ddc.moph.go.th/uploads/files/1532720201028081350.pdf. (In Thai)
Park, Y., Patwardhan, S., Visweswariah, K., & Gates, S. C. (2008, September 22-26). An Empirical Analysis of Word Error Rate and Keyword Error Rate. Interspeech 2008, 2070–2073. https://doi.org/10.21437/Interspeech.2008-537.
Phyathai Hospital. (2020). Slurred Speech, Difficulty Speaking, Slow Speech: Warning Signs that Require Urgent Treatment. https://www.phyathai.com/th/article/1758-พูดไม่ชัด_พูดไม่ออก_พูด. (In Thai)
Rukkhun Home Care. (n.d.). Aphasia - Speech Difficulties and Language Impairment in the Elderly. https://rukkhunhealth.com/blog/aphasia-in-elderly. (In Thai)
Schindel, D., Mandl, L., Schilling, R., Meisel, A., & Schenk, L. (2022). Guideline Adherence in Speech and Language Therapy in Stroke Aftercare. Health Insurance Claims Data Analysis. PLOS ONE, 17(2), e0263397. https://doi.org/10.1371/journal.pone.0263397
Shannon, M. (2017). Optimizing Expected Word Error Rate via Sampling for Speech Recognition. ArXiv. https://doi.org/10.48550/arXiv.1706.02776.
Young, B. M., Holman, E. A., Cramer, S. C., Shah, S., Griessenauer, C. J., Patel, N., Lin, D. J., Gee, J., Moon, J., Schwertfeger, J., Jayaraman, A., Lee, R., Lansberg, M., Payne, J., Patten, C., Cramer, S. C., Holman, E. A., Agrawal, K., Kissela, B., … Falcone, G. J. (2023). Rehabilitation Therapy Doses Are Low After Stroke and Predicted by Clinical Factors. Stroke, 54(3), 831–839. https://doi.org/10.1161/STROKEAHA.122.041098.
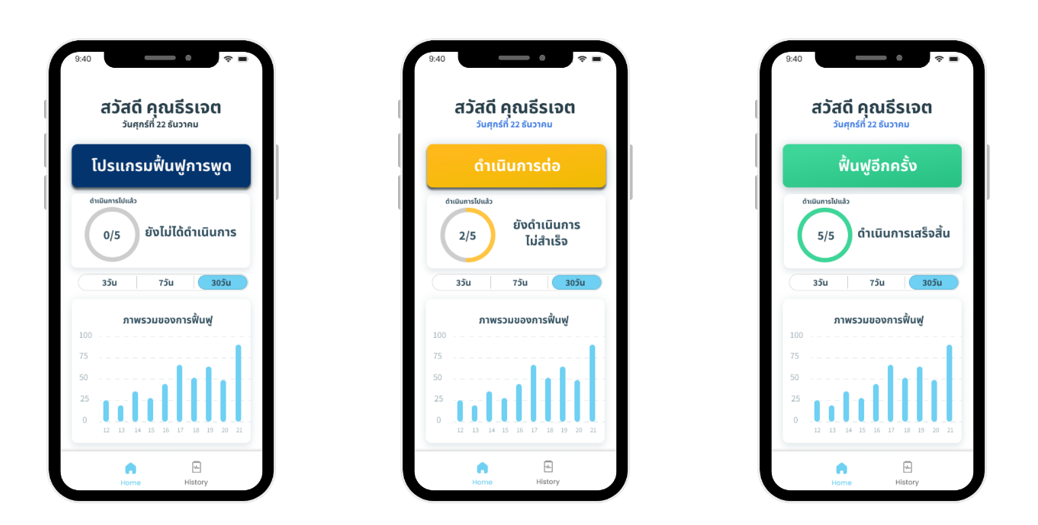
Downloads
Published
How to Cite
Issue
Section
Categories
License
Copyright (c) 2025 Journal of Computer and Creative Technology

This work is licensed under a Creative Commons Attribution-NonCommercial-NoDerivatives 4.0 International License.